In the digital age, the way we interact with our devices is undergoing a profound transformation. Voice and speech recognition technology, once a figment of science fiction, has now become an integral part of our daily lives. From smartphones that respond to our commands to smart homes that understand our preferences, the applications of this technology are expanding at an unprecedented pace.
The Rise of Voice-Activated Devices
The proliferation of voice-activated devices is a testament to the technology’s growing sophistication. According to a report by Statista, the number of digital voice assistants in use worldwide is projected to reach 8.4 billion units by 2024, which is more than the world’s population. This surge is driven by the convenience and efficiency that voice-activated systems offer, allowing for hands-free operation and providing assistance without the need for physical interaction.
Importance of Accuracy in Voice and Speech Recognition
At the heart of these systems lies the ability to accurately recognize and process human speech. The effectiveness of voice recognition technology hinges on its accuracy. It’s not just about understanding the words being spoken, but also grasping the context, the speaker’s intent, and the subtle nuances of language. This accuracy is crucial, as even a minor error can lead to a misunderstanding or a malfunction, disrupting the user experience.
The Evolution of Speech Recognition Accuracy
To illustrate the evolution of speech recognition accuracy, consider the following table that outlines the advancements over the years:
Year | Milestone | Accuracy | Impact |
---|---|---|---|
1995 | Early voice recognition systems | ~60% | Limited use in practical applications |
2000 | Introduction of machine learning | ~70% | Improved performance with basic commands |
2010 | Deep learning techniques applied | ~80% | Enhanced context understanding |
2015 | AI integration and neural networks | ~90% | Near-human accuracy in controlled environments |
2020 | Advanced AI algorithms | ~95%+ | Real-world application with complex interactions |
As the table suggests, the journey from rudimentary systems to advanced AI-powered solutions has been marked by significant milestones. Each leap in accuracy has opened new doors for application and innovation, leading us to today’s smart devices that can understand different dialects, accents, and even multiple languages with remarkable precision.
The integration of Artificial Intelligence (AI) into voice and speech recognition systems has been pivotal in this evolution. AI doesn’t just provide raw processing power; it brings a level of cognitive understanding that allows devices to learn from interactions, adapt to users’ speech patterns, and improve over time. This self-learning capability is what sets modern voice recognition systems apart and is the cornerstone of the technology’s future development.
As we stand on the brink of what AI can achieve in voice and speech recognition, it’s clear that the potential applications are as limitless as our ability to innovate. The following sections will delve deeper into how AI is revolutionizing voice testing, the challenges it faces, and the solutions it offers, paving the way for a future where technology understands us as well as we understand each other.
The AI Revolution in Voice Testing
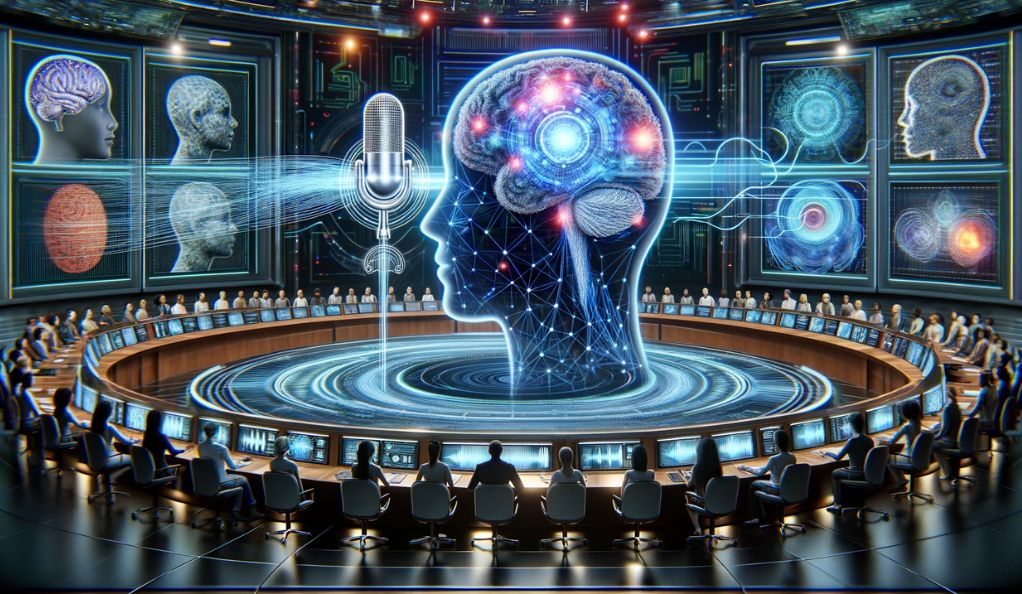
The advent of Artificial Intelligence (AI) has catalyzed a paradigm shift in the field of voice and speech recognition testing. AI is not just a tool but a transformative force that is reshaping how we approach quality assurance for voice-activated systems.
How AI is Changing the Landscape of Voice Quality Assurance
AI’s impact on voice quality assurance is multifaceted. Traditional testing methods often relied on manual processes, which were not only time-consuming but also prone to human error. With AI, the game has changed. Automated testing powered by AI can simulate a vast array of real-world conditions, from noisy environments to a multitude of accents, providing a level of thoroughness that is humanly unattainable.
AI Algorithms in Detecting and Learning Speech Patterns
AI algorithms are at the forefront of this revolution. They are designed to detect, analyze, and learn from speech patterns. This learning capability is crucial for handling the variability in human speech. AI systems use a variety of techniques, including:
- Natural Language Processing (NLP): To understand the content and intent behind spoken words.
- Machine Learning (ML): To identify patterns and improve recognition with exposure to more data.
- Deep Learning (DL): To perform more complex tasks like sentiment analysis and contextual understanding.
The Synergy of AI and Human Oversight
While AI brings efficiency and scalability to the testing process, human oversight remains crucial. AI algorithms are initially trained by linguists and voice experts to understand the intricacies of language. This collaboration ensures that the AI systems are calibrated to the highest standards of accuracy before being deployed for automated testing.
Challenges in Voice Recognition Testing
Voice recognition technology, despite its advancements, faces a myriad of challenges that can impede its performance. These challenges are not just technical but also linguistic and environmental. Addressing them is critical to ensuring that voice-activated systems can function effectively in the diverse and unpredictable real world.
Variability in Speech: Accents, Dialects, and Intonations
One of the most significant hurdles in voice recognition is the inherent variability in human speech. Accents, dialects, and intonations can vary dramatically, even within the same language. For instance, the word “water” can be pronounced differently across various English-speaking regions, which can confuse a voice recognition system not adequately trained to recognize these variations.
Background Noise and Its Impact on Recognition Accuracy
Another major challenge is background noise. In real-world scenarios, voice-activated devices must contend with a plethora of sounds—from the hum of a refrigerator to the chatter in a crowded room. These noises can mask speech signals, leading to decreased recognition accuracy.
Addressing the Challenges: A Multifaceted Approach
To overcome these challenges, a multifaceted approach is necessary. This includes:
- Acoustic Modeling: Creating models that can accurately represent the acoustic characteristics of speech within noisy environments.
- Language Modeling: Developing comprehensive language models that account for variations in dialects and accents.
- Robust Testing Protocols: Implementing testing protocols that cover a wide range of speech scenarios and environmental conditions.
The Role of AI in Overcoming Testing Challenges
AI, with its ability to learn and adapt, is uniquely positioned to tackle these challenges. By employing advanced algorithms, AI can analyze vast datasets of speech, learn from the variability, and improve its recognition capabilities. For example, deep learning models can filter out background noise, while NLP can discern meaning from complex speech patterns.
AI-Driven Solutions for Enhanced Testing
The challenges of voice recognition testing are formidable, but AI-driven solutions are paving the way for a new era of enhanced voice quality assurance. These solutions leverage the power of AI to not only address the current limitations but also to anticipate future demands.
Machine Learning Models for Noise Cancellation and Pattern Recognition
One of the most significant advancements in AI-driven voice testing is the development of sophisticated machine learning models that excel at noise cancellation and speech pattern recognition. These models are trained on diverse datasets that include various speech nuances and background noise scenarios, enabling them to distinguish between the speaker’s voice and unwanted sounds effectively.
Deep Learning for Contextual Understanding
Deep learning, a subset of machine learning, takes this a step further by providing contextual understanding. This is particularly important for voice recognition systems as it allows them to interpret the meaning behind words, which can be influenced by context. For example, the phrase “Let’s play” could refer to playing music or starting a game, depending on the preceding conversation.
Case Studies: Successful AI Implementations in Voice Testing
Several case studies highlight the success of AI in voice recognition testing. Companies like Google and Amazon have made significant strides in this area, with their virtual assistants now able to understand a wide range of commands in noisy environments. This is largely due to their investment in AI and machine learning, which has enabled them to develop algorithms that can learn from user interactions and improve over time.
The Impact of AI on Testing Protocols
The integration of AI has also led to the evolution of testing protocols. Traditional testing methods are being supplemented with AI-driven simulations that can create a multitude of virtual environments and scenarios, from a quiet office to a bustling street. This allows for more comprehensive testing without the need for extensive physical setups.
AI Techniques and Their Impact on Voice Testing
AI Technique | Application in Voice Testing | Impact |
---|---|---|
Noise Cancellation Algorithms | Filtering out background sounds | Higher accuracy in noisy environments |
Speech Pattern Recognition | Identifying unique speech characteristics | Better recognition of accents and dialects |
Contextual Analysis | Understanding the context of commands | Improved interpretation of user intent |
Automated Testing Protocols | Simulating diverse scenarios | More efficient and thorough testing processes |
The Future of AI in Voice Testing
The future of AI in voice testing looks promising. With ongoing advancements in AI and machine learning, we can expect voice recognition systems to become even more sophisticated. The goal is to achieve a level of understanding that is indistinguishable from human interaction, where devices can not only recognize speech but also understand the speaker’s emotions and respond appropriately.
Testing Across Different Languages and Accents
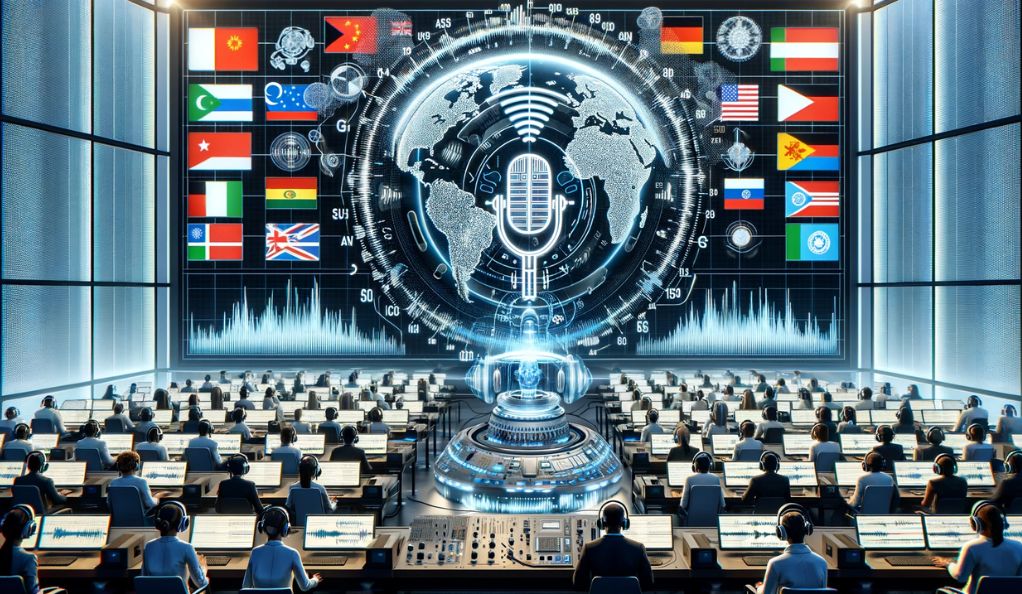
The global nature of technology today demands that voice recognition systems are not only accurate in one language but across many. This section explores the complexities of multi-language support in AI testing and the techniques used to train AI on diverse linguistic datasets.
The Complexity of Multi-Language Support in AI Testing
Voice recognition systems must navigate a labyrinth of linguistic complexities to serve a global user base. Each language comes with its own set of rules, accents, and idiomatic expressions, which can vary widely even within the same country. For instance, the nuances between British, American, and Australian English are substantial enough to affect recognition accuracy.
Techniques for Training AI on Diverse Linguistic Datasets
To ensure that AI systems can understand and process multiple languages and accents, developers employ several techniques:
- Diverse Data Collection: Gathering voice data from speakers of different languages and accents to create a rich, varied dataset.
- Transfer Learning: Applying knowledge gained from one language to improve performance in another.
- Accent Adaptation: Training AI models to recognize and adapt to the unique characteristics of various accents.
Challenges and Solutions in Multi-Language Testing
Despite the best efforts, multi-language testing presents challenges. One significant issue is the scarcity of data for less common languages or dialects. To combat this, AI systems can use synthetic data generation to fill the gaps, creating artificial speech samples that mimic the target language or accent.
The Role of Synthetic Voices in Testing Scenarios
As the demand for comprehensive voice recognition testing grows, the use of synthetic voices has become increasingly important. Synthetic voices are artificially generated sounds that mimic human speech, and they play a crucial role in creating diverse and scalable testing environments.
Generating Synthetic Speech for Comprehensive Testing
Synthetic speech generation involves creating a database of spoken words, phrases, and sentences that can be used to test voice recognition systems. This is particularly useful for languages or dialects where recorded speech data is scarce. Advanced text-to-speech (TTS) technologies powered by AI can now produce speech that closely resembles natural human intonation and rhythm.
Balancing Synthetic and Natural Speech Inputs in Test Cases
While synthetic voices are invaluable for testing, they must be balanced with natural speech inputs to ensure that voice recognition systems are exposed to the full spectrum of human speech variability. The following table illustrates how synthetic and natural speech inputs can be integrated into the testing process:
Input Type | Use Case | Advantages | Limitations |
---|---|---|---|
Synthetic Speech | Testing system response to uncommon phrases | Controlled, consistent, and can be generated on demand | May lack the nuances of natural speech |
Natural Speech | Evaluating system performance with real-world data | Contains the natural variability and complexity of human speech | Collection can be time-consuming and less controlled |
The Advantages of Synthetic Voices in Testing
The advantages of using synthetic voices in testing are manifold:
- Scalability: Synthetic voices can be generated in large quantities, allowing for extensive testing across various scenarios without the need for a large number of human speakers.
- Consistency: They provide a consistent baseline for testing, which is essential for benchmarking and comparative analysis.
- Customization: Synthetic voices can be tailored to specific needs, such as particular accents, ages, or languages that might be underrepresented in available speech data.
The Future of Synthetic Speech in Voice Testing
Looking ahead, the role of synthetic speech in voice testing is set to expand. With the continuous improvement of AI-generated voices, the line between synthetic and natural speech is becoming increasingly blurred. This will allow for even more nuanced and sophisticated testing, ensuring that voice recognition systems are prepared for the complexities of human communication.
Future Trends in AI and Voice Recognition Testing
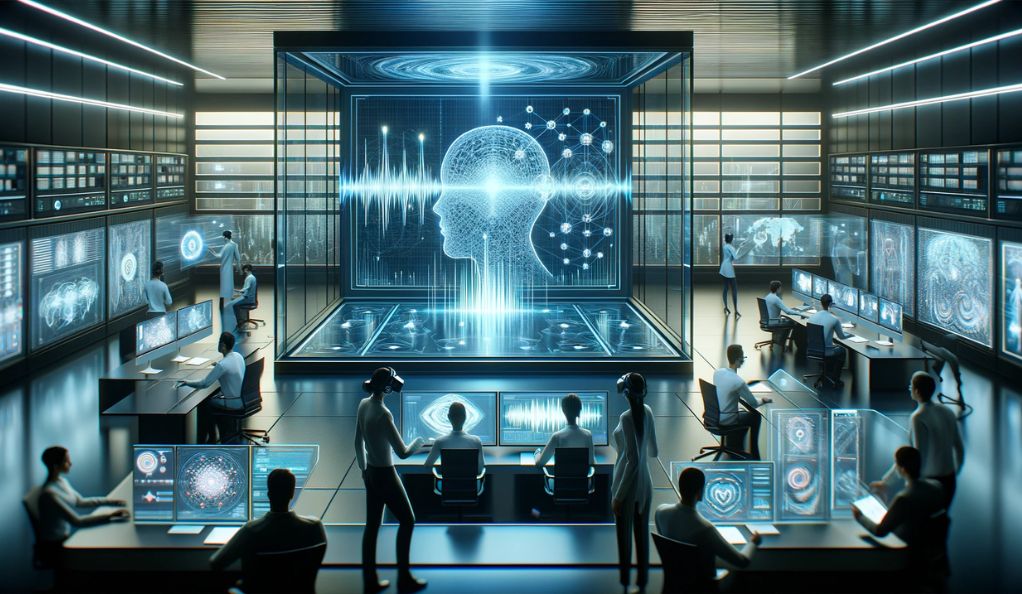
As we look to the horizon of voice recognition technology, several emerging trends are set to redefine the landscape of AI-driven testing. These trends not only promise to enhance the capabilities of voice recognition systems but also to streamline the testing process itself.
Predictive Analytics in Voice Quality Assurance
Predictive analytics is one of the most exciting developments in the field of AI and voice recognition testing. By analyzing large datasets, AI can predict potential issues before they arise, allowing developers to proactively make adjustments. This anticipatory approach to quality assurance can significantly reduce the time and resources spent on testing.
The Potential of AI in Personalized Voice Interactions
Another trend is the personalization of voice interactions. AI systems are beginning to use voice data to tailor responses to individual users, taking into account their preferences and usage patterns. This level of personalization requires rigorous testing to ensure that the systems can adapt to individual users without compromising privacy or security.
Cross-Platform Integration and Consistency
The integration of voice recognition across multiple platforms presents another challenge. As users increasingly expect a seamless experience across their devices, from smartphones to smart home systems, testing must ensure consistency in recognition and response, regardless of the platform.
Ethical Considerations and Privacy in Voice Testing
As AI continues to advance the capabilities of voice and speech recognition technologies, it is imperative to address the ethical considerations and privacy concerns that accompany these developments. The collection and use of voice data for testing and improving AI systems raise significant questions about user consent, data security, and the potential for misuse.
Ensuring User Data Protection During the Testing Process
Protecting user data during the testing process is not just a technical challenge but a moral obligation. Companies must implement robust data protection measures to ensure that voice data is anonymized and secure from unauthorized access. This involves encryption, secure data storage solutions, and strict access controls.
Ethical AI: Balancing Innovation with Responsibility
The concept of Ethical AI is gaining traction, emphasizing the need for AI systems to be designed and tested with ethical principles in mind. This includes transparency, accountability, and fairness in AI interactions. Developers must ensure that AI systems do not perpetuate biases or discrimination, which requires diverse datasets and unbiased algorithms.
Case Studies: Ethical Practices in Voice Testing
Several companies are leading by example, implementing best practices in ethical AI and voice testing. For instance, some have established independent ethics boards to oversee AI development, while others publish transparency reports detailing their use of voice data.
The Future of Ethical Voice Testing
Looking forward, the field of voice testing is likely to see increased regulation and standardization around ethical practices. This could include industry-wide standards for data protection, as well as international agreements on the ethical use of AI.
Conclusion: The Path Forward for AI in Voice and Speech Recognition Testing
In conclusion, the integration of AI into voice and speech recognition testing heralds a transformative era for human-computer interaction, marked by significant strides in accuracy, efficiency, and the ability to navigate the complexities of human language. As we look to the future, the promise of AI is not only in its technological prowess but also in its potential to create more inclusive and accessible communication tools. However, this bright future hinges on our collective commitment to ethical development and privacy safeguards, ensuring that advancements in AI empower and protect users across the globe. The path forward is one of responsible innovation, where the benefits of AI are realized without compromising the values we hold dear.