In the rapidly evolving world of technology, generative artificial intelligence (AI) stands out as a groundbreaking development. This form of AI, which includes technologies like deep learning and neural networks, goes beyond analyzing data—it creates. From generating realistic images to synthesizing human-like text, the capabilities of generative AI are expanding the boundaries of what machines can do.
Understanding the Interaction
The importance of data privacy in the AI landscape is paramount. As we entrust more personal information to digital platforms, the risks of data breaches and misuse escalate. With AI’s integration into various sectors, such as healthcare and finance, the potential consequences of compromised data privacy become increasingly significant.
Setting the Stage
To fully understand the interaction between generative AI and data privacy, it’s essential to explore several key areas:
- Data Sensitivity and AI: Generative AI systems can potentially expose sensitive data, either through the data they generate or through the data they are trained on. For instance, an AI model trained on medical records must be designed to protect patient confidentiality.
- Consent and Data Use: In many cases, the data used to train generative AI models is gathered from users who may not fully understand how their information will be used. This raises questions about informed consent and ethical data use.
- Data Anonymization: While anonymizing data can help protect privacy, it’s challenging to implement effectively in AI systems. AI’s ability to cross-reference data can sometimes re-identify anonymized information.
- Balancing Innovation with Privacy: There’s a delicate balance to be struck between leveraging the power of AI and ensuring that individual privacy rights are respected. Finding this balance is crucial for the sustainable development of AI technologies.
Understanding Data Privacy Laws in the AI Era
The landscape of data privacy has become increasingly complex with the advent of generative AI. To navigate this terrain, it’s crucial to understand the global legal frameworks that govern data protection and how they interact with AI technologies. Here, we explore some of the key data privacy laws and their implications for AI.
The General Data Protection Regulation (GDPR) – Europe
The GDPR, implemented in the European Union in 2018, is one of the most comprehensive data protection regulations globally. It sets stringent requirements for data processing and grants individuals substantial control over their personal data. Key aspects relevant to AI include:
- Consent for Data Processing: The GDPR requires explicit consent for processing personal data, which impacts how AI systems collect and use data.
- Right to Explanation: Individuals have the right to an explanation of decisions made by automated systems, which is a significant consideration for AI transparency.
- Data Minimization: The principle of data minimization under GDPR mandates that only necessary data for a specific purpose be collected, posing a challenge for data-intensive AI models.
The California Consumer Privacy Act (CCPA) – United States
The CCPA, which came into effect in 2020, is one of the United States’ most robust data privacy laws. It gives California residents rights over their personal information, including:
- Right to Know: Consumers can request to know what personal information a business collects, uses, shares, or sells.
- Right to Delete: Consumers can ask businesses to delete their personal information.
- Opt-Out Right for Data Sales: Consumers have the right to opt-out of the sale of their personal information, a crucial aspect for AI systems that rely on data monetization.
Generative AI developers and users must navigate these legal requirements carefully. The laws set the foundation for ethical AI development, ensuring that personal data is used responsibly and transparently. However, they also pose challenges, particularly in terms of the volume of data needed for AI systems and the need for clarity in how AI decisions are made.
As AI continues to evolve, so too will the legal frameworks governing it. It’s essential for stakeholders in the AI field to stay informed about these changes and actively engage in shaping policies that balance innovation with privacy protection.
Generative AI: Risks and Concerns for Data Privacy
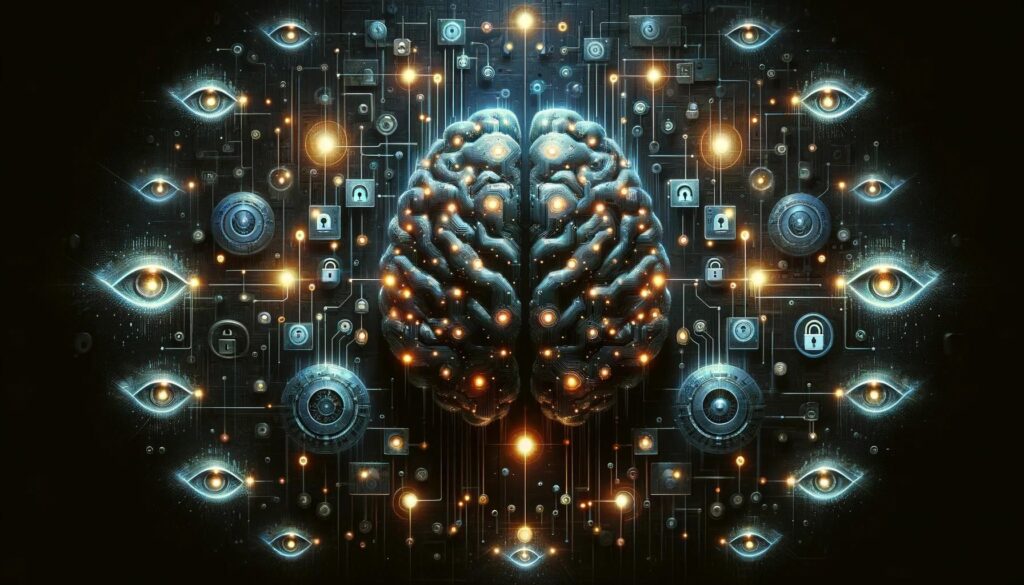
The burgeoning field of generative AI, while offering remarkable capabilities, also introduces significant challenges in terms of data privacy. These advanced systems, adept at creating new content from existing datasets, pose risks in exposing sensitive information, misusing data, and creating privacy dilemmas. This section highlights the primary risks associated with generative AI and their implications for data privacy.
Potential Risks of AI in Terms of Data Breaches and Misuse
- Data Breaches: Generative AI systems, processing extensive datasets, can become prime targets for cyberattacks. Such breaches could lead to large-scale exposure of sensitive data.
- Misuse of Data: There is a risk that generative AI could be employed to create deceptive or harmful content, like deepfakes. This misuse poses significant threats to individual privacy and public trust.
- Inadvertent Data Exposure: AI models, especially in machine learning, may unintentionally reveal sensitive information. For example, an AI trained on healthcare records might inadvertently include personal health information in its outputs.
Understanding the Privacy Implications Through Examples
- Facial Recognition Systems: These AI-powered systems can recognize individuals without consent, raising critical privacy concerns about unauthorized surveillance.
- Text Generation Models: Advanced models like GPT-3 generate realistic text based on user inputs, which could unintentionally incorporate or reveal personal or sensitive data.
Risk Type | Example | Mitigation Strategy |
---|---|---|
Data Breaches | Hacking of AI systems | Implement robust cybersecurity measures |
Misuse of Data | Use in creating deepfakes | Develop ethical guidelines for AI usage |
Inadvertent Exposure | Leakage of health data in outputs | Employ advanced data anonymization techniques |
These risks underscore the critical need for robust measures to protect data privacy in the context of generative AI. The focus is not only on safeguarding data from external threats but also ensuring that AI systems are inherently designed with privacy considerations. Subsequent sections will delve into the ethical implications of AI in data handling, the technological measures for privacy protection, and the pivotal role of regulation and compliance in addressing these risks.
Ethical Implications of AI in Data Handling
The integration of generative AI into various sectors brings with it not just technological challenges, but also complex ethical considerations, particularly in terms of data handling. This section explores the ethical dilemmas and responsibilities that arise in the context of AI data collection, processing, and usage.
Ethical Dilemmas in AI Data Collection and Processing
- Informed Consent: A significant ethical issue in AI revolves around obtaining informed consent from individuals whose data is used. Many users are unaware of how their data is being collected, processed, and utilized by AI systems.
- Bias and Fairness: AI systems can perpetuate and even amplify biases present in their training data, leading to unfair outcomes. Ensuring fairness in AI models is a crucial ethical concern.
- Transparency and Accountability: There is a growing demand for transparency in AI algorithms and their decision-making processes. Accountability for decisions made by AI systems is a key ethical issue, especially when these decisions impact individuals’ lives.
Role of Ethics in AI Development and Deployment
- Developing Ethical Guidelines: Establishing ethical guidelines for AI development is crucial. These guidelines should address data privacy, bias, transparency, and accountability.
- Ethical Audits and Assessments: Regular ethical audits and impact assessments can help identify potential ethical issues in AI systems and guide corrective measures.
The ethical implications of AI in data handling extend beyond legal compliance. They involve a commitment to creating AI systems that are not only technologically advanced but also ethically sound and respectful of individual rights and societal norms. The next sections will explore technological measures for protecting privacy, the role of regulation and compliance, and emerging trends in AI and privacy.
Technological Measures for Protecting Privacy in Generative AI
As the capabilities of generative AI continue to grow, so does the need for robust technological measures to ensure data privacy. This section explores the innovations and strategies employed in AI to enhance privacy protection, focusing on encryption, anonymization, and other advanced techniques.
Innovations in AI that Enhance Data Privacy
- Differential Privacy: This technique adds ‘noise’ to the data or the AI’s outputs, making it difficult to identify individual data points. It’s increasingly used in AI to protect user data while providing valuable insights.
- Homomorphic Encryption: This form of encryption allows data to be processed while still encrypted, significantly enhancing data security. AI systems can analyze encrypted data without ever decrypting it, thus safeguarding sensitive information.
- Federated Learning: Instead of centralizing data, federated learning allows AI models to be trained on decentralized data. This approach minimizes the risk of data breaches and enhances privacy by keeping sensitive data on local devices.
Use of Encryption, Anonymization, and Other Techniques.

- Encryption: This is the process of encoding data so that only authorized parties can access it. In the context of AI, encryption is used to secure data both in transit (as it moves across networks) and at rest (when stored on servers). This prevents unauthorized access and ensures that data remains confidential. Advanced encryption methods, like homomorphic encryption, allow data to be processed without ever needing to be decrypted, offering an additional layer of security.
- Anonymization: Anonymization involves stripping away personally identifiable information from a dataset. The goal is to modify the data so that individuals cannot be readily identified, directly or indirectly, by the information in the dataset. This is particularly important in AI, where large datasets might contain sensitive personal information. Proper anonymization helps in maintaining privacy while still allowing the data to be useful for analysis.
- Other Techniques: Apart from encryption and anonymization, there are other methods employed to safeguard data privacy, such as:
- Data Masking: This involves obscuring specific data within a database to protect it. For instance, a portion of a Social Security number or credit card number might be masked in a dataset used for analysis.
- Secure Multi-party Computation (SMC): This technique allows multiple parties to input data into an AI algorithm without revealing their actual data to each other. It’s useful in scenarios where data privacy is paramount but collaboration is necessary.
- Data Minimization: This is the practice of only collecting and processing the minimum amount of data necessary for a specific purpose. It’s a principle of data protection that aims to limit the amount of data an entity holds, thereby reducing the risk of data breaches and privacy violations.
Each of these methods plays a crucial role in ensuring the security and privacy of data used in AI systems. As AI technology continues to advance and handle more sensitive data, the importance and sophistication of these techniques are likely to grow.
Privacy Protection Technologies in AI
- Differential Privacy
- Description: Adds noise to data or outputs.
- Privacy Benefit: Protects individual data points.
- Homomorphic Encryption
- Description: Allows processing of encrypted data.
- Privacy Benefit: Secures data during analysis.
- Federated Learning
- Description: Trains AI on decentralized data.
- Privacy Benefit: Minimizes central data breach risks.
- Encryption
- Description: Secures data against unauthorized access.
- Privacy Benefit: Safeguards data in transit and at rest.
- Anonymization
- Description: Removes identifying details from data.
- Privacy Benefit: Protects individual identities.
- Secure Multi-party Computation
- Description: Joint computation with private inputs.
- Privacy Benefit: Facilitates sensitive data sharing.
These technological measures are essential in the development and deployment of generative AI systems, ensuring that user privacy is not compromised. As AI continues to integrate into various sectors, the implementation of these privacy-enhancing technologies becomes increasingly critical. The upcoming sections will discuss the role of regulation and compliance, as well as future trends in AI and privacy.
The Role of Regulation and Compliance in AI Data Privacy
As generative AI continues to make strides, the role of regulation and compliance becomes increasingly crucial in ensuring data privacy. This section examines how regulations shape AI development and the compliance challenges companies face when using generative AI technologies.
How Regulations Can Shape AI Development for Privacy Protection
Regulations play a pivotal role in guiding the ethical and responsible use of AI. They set the standards for privacy, security, and data usage, influencing how AI technologies are designed and deployed. Key aspects include:
- Data Protection Regulations: Laws like the GDPR and CCPA set the bar for how personal data should be handled, compelling AI developers to incorporate privacy by design.
- AI-Specific Regulations: Some jurisdictions are beginning to introduce AI-specific regulations that address the unique challenges posed by AI technologies, including transparency, accountability, and ethical considerations.
- Cross-Border Data Flow Restrictions: Regulations often include provisions on cross-border data transfers, impacting how global AI systems operate and manage data privacy across different legal jurisdictions.
Compliance Challenges for Companies Using Generative AI
Companies leveraging generative AI face several compliance challenges:
- Keeping Up with Evolving Regulations: As AI technology and data privacy laws evolve, companies must continuously update their practices to remain compliant.
- Balancing Innovation with Compliance: Finding a balance between harnessing the capabilities of AI and adhering to stringent privacy regulations can be challenging.
- Global Data Privacy Considerations: Companies operating internationally must navigate a complex web of data privacy laws, which can vary significantly from one region to another.
Regulation Impact and Compliance Strategies in AI
- Data Protection Regulations
- Impact on AI: Privacy by design in AI.
- Compliance Strategy: Incorporate privacy features in AI development.
- AI-Specific Regulations
- Impact on AI: Accountability in AI decisions.
- Compliance Strategy: Develop transparent AI systems and decision-making processes.
- Cross-Border Data Transfers
- Impact on AI: Data flow management in global AI systems.
- Compliance Strategy: Implement robust data governance policies.
These regulatory aspects and their corresponding impacts and strategies highlight the complexity of integrating AI within existing and evolving legal frameworks. Adhering to these regulations is crucial for ensuring that AI systems are both effective and compliant with global data privacy standards. The sections to follow will further delve into these aspects, offering deeper insights into the evolving relationship between AI, regulation, and privacy.
Future Trends: AI, Privacy, and Beyond
The intersection of generative AI and data privacy is not static; it is a rapidly evolving space with emerging trends that are shaping the future of technology, law, and societal norms. This section explores these trends and their potential impact on privacy.
Emerging Trends in AI
- Increased Use of Synthetic Data: To mitigate privacy concerns, there’s a growing trend towards the use of synthetic data – artificially generated data that mimics real datasets but does not contain real personal information. This approach can help in training AI models while preserving privacy.
- Advancement in Privacy-Preserving Technologies: Technologies like differential privacy and federated learning are advancing, offering more sophisticated ways to protect data privacy in AI applications.
- Greater Focus on Ethical AI: There’s an increasing emphasis on developing AI ethically, which includes considerations for privacy, fairness, transparency, and accountability.
Potential Impact on Privacy
- Enhanced Data Security: As privacy-preserving technologies become more advanced, we can expect a higher level of data security in AI systems.
- Shifts in Regulatory Frameworks: As AI technology evolves, so too will the legal frameworks governing it. We may see more AI-specific regulations that address the unique challenges of AI and privacy.
- Increased Public Awareness and Engagement: As the public becomes more aware of AI and privacy issues, there will likely be greater demand for transparency and ethical considerations in AI development.
Future Trends and Their Implications for AI and Privacy
- Use of Synthetic Data
- Description: Generating artificial data for AI training.
- Implication for Privacy: Reduces the risk of exposing real personal data.
- Advancement in Privacy Technologies
- Description: Developing more sophisticated privacy methods.
- Implication for Privacy: Enhances the ability to protect data privacy.
- Focus on Ethical AI
- Description: Emphasizing ethical considerations in AI.
- Implication for Privacy: Leads to more responsible and transparent AI practices.
These trends suggest a future where AI and privacy can coexist more harmoniously. As technology progresses, coupled with shifts in societal and regulatory perspectives, there is likely to be a better balance between leveraging the capabilities of AI and protecting individual privacy. The conclusion of the article will further summarize this balance between AI innovation and data privacy, providing final thoughts on the evolving landscape.
Striking a Balance Between AI Innovation and Data Privacy
As we delve into the intersection of generative AI and data privacy, it becomes evident that the path forward is a delicate balancing act. On one hand, AI’s rapid advancements offer groundbreaking potential across various sectors, promising efficiency, innovation, and even solutions to complex societal challenges. On the other hand, these advancements raise substantial privacy concerns that cannot be overlooked. Ensuring that AI development and deployment are guided by robust privacy protections, ethical standards, and transparent practices is crucial. It’s about creating a synergy where technological innovation flourishes without compromising the sanctity of individual privacy rights.
Looking ahead, the dynamic between AI and privacy will continue to evolve. As technology advances, so will the mechanisms and strategies to safeguard privacy. This ongoing progression demands adaptability, foresight, and a collaborative approach among technologists, policymakers, and the public. The ultimate goal is a future where the potentials of AI are harnessed responsibly, ensuring that privacy considerations are not just an afterthought but a foundational aspect of innovation. In this way, generative AI can fulfill its promise as a transformative force in a world where privacy is respected and protected.