In recent years, generative artificial intelligence (AI) has emerged as a groundbreaking technology, reshaping the landscape of numerous industries with its innovative capabilities. At its core, generative AI refers to algorithms that can learn from a vast array of data inputs and then generate new, original content or solutions that were not explicitly programmed. This technology spans a wide array of applications, from creating realistic images and text to solving complex scientific problems.
The Essence of Generative AI
Generative AI, leveraging advanced machine learning models like neural networks, learns from extensive datasets to mimic complex patterns. Key forms include Generative Adversarial Networks (GANs), where two networks collaboratively generate and evaluate content; Variational Autoencoders (VAEs), used in image and voice generation for data compression and reconstruction; and Transformer Models, renowned for their prowess in natural language processing, powering sophisticated text-generation systems. These technologies exemplify generative AI’s diverse applications.
Sector | Application |
Art and Design | Pushing creative boundaries with AI-generated elements |
Healthcare | Drug discovery and personalized medicine solutions |
Finance | Revolutionizing risk assessment and decision-making |
Entertainment | Facilitating content creation in music, books, and film |
Scientific Research | Accelerating research with hypothesis generation |
The Ethical Implications
While the advancements in generative AI are undeniably impressive, they come with a host of ethical considerations. Issues like data privacy, potential biases in AI algorithms, and the balance between innovation and regulatory controls are at the forefront of the ethical debate. As we delve deeper into this technology, understanding and addressing these ethical challenges becomes paramount to ensure that generative AI serves humanity positively and responsibly.
Understanding Data Privacy in Generative AI
Comprehending data privacy in generative AI is essential. It encompasses safeguarding sensitive data and maintaining ethical usage, vital for trust and compliance.
The Significance of Data in AI Training
Data serves as the lifeblood of generative AI. These systems require extensive datasets to learn and improve. The quality, variety, and volume of the data directly influence the AI’s performance and capability. In training, these algorithms sift through vast amounts of information, learning patterns and nuances that enable them to generate new, original content or solutions. From textual data for language models to images for visual recognition systems, the diversity of data types is vast and continues to expand as AI capabilities grow.
Privacy Concerns and Potential Risks
However, this reliance on large datasets raises significant privacy concerns. The primary risk lies in the source of this data. Often, it encompasses personal information, which, if mishandled, can lead to privacy breaches. Additionally, there’s the challenge of ‘informed consent,’ where individuals might be unaware that their data is being used to train AI systems. Another risk involves the unintentional embedding of sensitive information within the AI model itself, which could be extracted by malicious actors. As generative AI becomes more integrated into our lives, ensuring the privacy and security of the underlying data becomes a critical concern that demands robust solutions and vigilant oversight.
Confronting Bias in AI Algorithms
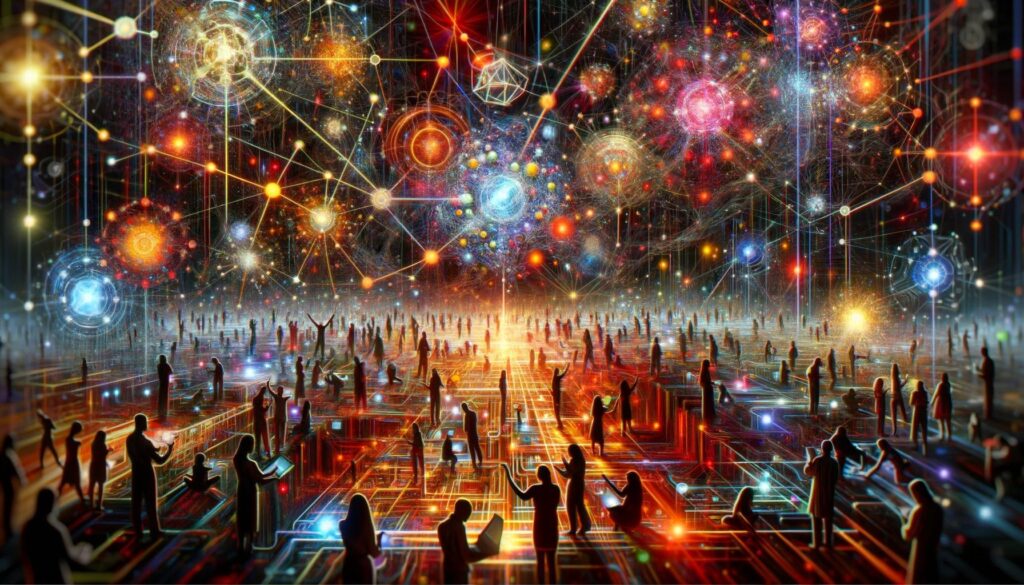
Addressing AI algorithmic bias is paramount. It involves identifying and rectifying discriminatory patterns in AI systems, promoting fairness, and enhancing trust in artificial intelligence.
How Bias Enters AI Models
Bias in AI models often originates from the data used for training. If the data set reflects historical prejudices or lacks diversity, the AI is likely to inherit these biases. For example, if a facial recognition system is trained predominantly on images of individuals from a single racial group, it may struggle to accurately recognize faces from other racial groups. Similarly, language processing algorithms can perpetuate gender biases if trained on text that reflects stereotypical roles. These biases are not always intentional but can arise from overlooked limitations in data collection and curation processes.
The Impact of Biased AI on Society
The consequences of biased AI algorithms extend far beyond technical inaccuracies. They have profound societal implications. In areas like law enforcement, biased facial recognition can lead to wrongful identification and unfair treatment. In hiring, AI-driven applicant screening tools may inadvertently favor certain groups over others. Such biases in AI can reinforce existing societal inequalities and discrimination, making it crucial for developers to actively work on identifying and mitigating biases in AI systems. This is not just a technical challenge but a moral imperative to ensure equitable and fair use of AI in society.
Balancing Innovation with Regulation
Striking the balance between innovation and regulation is crucial. It requires fostering technological advancement while ensuring responsible oversight to maintain ethical and societal standards.
The Need for Regulatory Frameworks
As generative AI continues to evolve, the need for comprehensive regulatory frameworks becomes increasingly evident. These frameworks are essential not only for mitigating risks associated with AI, such as privacy breaches and biases, but also for fostering an environment where innovation can thrive responsibly. Effective regulation should strike a balance between safeguarding public interests and encouraging technological advancements. This involves setting clear guidelines on data usage, ethical AI development practices, and ensuring accountability in AI-driven decisions.
Examples of AI Regulation Case Studies
Several instances highlight how various regions and sectors are approaching AI regulation:
- European Union’s AI Act: Proposed as a comprehensive legal framework for AI, it aims to address risks associated with AI systems and establish standards for high-risk applications.
- United States’ Algorithmic Accountability Act: This proposed legislation focuses on requiring companies to assess and correct algorithmic biases, particularly in critical areas like employment and criminal justice.
- Singapore’s Model AI Governance Framework: An initiative that provides detailed guidelines for the responsible deployment of AI, emphasizing transparency and fairness.
- China’s New Data Security Law: Enacted to regulate data processing activities, enhance data security, and protect the rights of individuals, reflecting a growing concern over AI and data governance.
AI and Human Collaboration in the Workforce
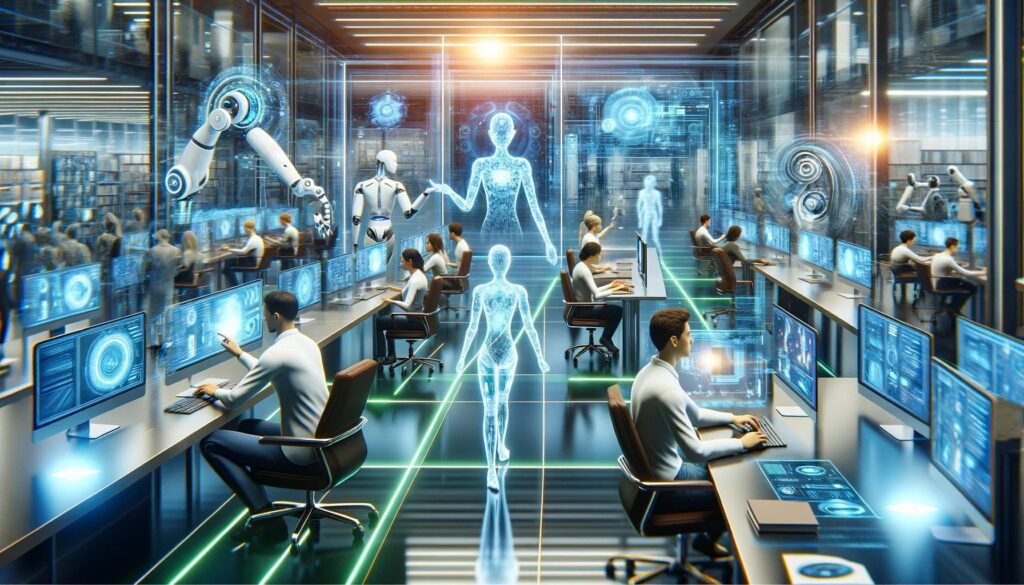
AI-human collaboration is shaping the workforce’s future. It entails optimizing the partnership between AI and human skills to enhance productivity, efficiency, and innovation in various industries.
AI’s Role in Job Displacement and Creation
The integration of AI into the workforce presents a dual narrative: while it leads to the displacement of certain jobs, particularly those involving repetitive or manual tasks, it also creates new opportunities in emerging fields. For instance, automation driven by AI has significantly reduced the need for manual labor in manufacturing and data processing. Conversely, this technological evolution is generating demand for jobs in AI development, data analysis, and AI ethics management. The key lies in understanding that AI does not merely replace jobs but rather shifts the nature of work, necessitating adaptation and reskilling in the workforce.
Ethical Considerations in AI-Assisted Workplaces
In workplaces augmented by AI, several ethical considerations arise:
- Fairness in Automation: Ensuring that automation does not disproportionately affect certain groups of workers.
- Transparency: Workers should be aware of how and why AI is used in their workplace, especially in decision-making processes.
- Reskilling and Education: Companies must invest in training programs to help employees adapt to new roles created by AI advancements.
- Worker Well-being: Monitoring the impact of AI on mental and physical health, especially in high-stress environments like algorithmic trading or high-speed manufacturing.
- Data Privacy: Safeguarding employee data used in AI systems, ensuring confidentiality and consent.
The Moral Responsibility of AI Developers
AI developers bear moral responsibility. They must prioritize ethical considerations, minimize harm, and design AI systems with accountability, ensuring technology serves society’s best interests.
Ethical Guidelines for AI Development
AI developers play a pivotal role in shaping the ethical landscape of AI technology. Adhering to ethical guidelines is crucial in this process:
- Non-maleficence: Avoid creating AI systems that can harm users or society.
- Justice: Ensure AI systems do not perpetuate or exacerbate social inequalities.
- Autonomy: Respect user autonomy, particularly in matters of privacy and consent.
- Transparency: Make the workings of AI systems as transparent and understandable as possible.
- Responsibility: Take accountability for the impacts of AI systems, both intended and unintended.
- Privacy Protection: Implement robust measures to safeguard user data against misuse or breaches.
- Inclusivity: Strive for diversity in AI development teams and data sets to reduce biases.
The Role of Transparency and Accountability
Transparency and accountability are essential in building trust between AI developers and the public. Transparency involves explaining how AI systems work and the logic behind their decisions, making it easier for users to understand and trust AI outputs. It also includes being open about the limitations and potential errors of AI systems. Accountability, on the other hand, pertains to developers taking responsibility for the outcomes of their AI systems, including addressing any negative impacts. This includes establishing mechanisms for feedback and redress for those affected by AI decisions. By prioritizing transparency and accountability, AI developers can foster a more ethical and trustworthy AI ecosystem.
Conclusion
In the rapidly evolving field of generative AI, developers face a profound moral responsibility. Adhering to ethical guidelines is essential; these include ensuring non-maleficence, promoting justice, respecting user autonomy, and guaranteeing privacy. Emphasizing transparency and accountability, developers must ensure that AI systems are understandable and that they responsibly address any societal impacts. This commitment is vital for fostering trust and ensuring that AI advancements align with ethical standards and contribute positively to society.