The manufacturing sector, known for embracing technological advancements, is now witnessing the profound impact of Artificial Intelligence (AI). AI in manufacturing extends beyond automation and robotics, focusing on efficiency, cost reduction, and product quality improvement. Predictive maintenance, powered by AI, plays a pivotal role by forecasting and preventing equipment failures, ensuring uninterrupted operations and reduced downtime.
The Shift Towards Smart Manufacturing
The adoption of AI in manufacturing signifies a shift towards ‘smart manufacturing’, where factories are more digitized, interconnected, and intelligent. This transition is driven by the need for greater efficiency and flexibility in production processes. AI-driven systems can analyze vast amounts of data, learning from patterns and anomalies to make real-time decisions that can significantly improve manufacturing operations.
Predictive Maintenance: A Cornerstone of AI Applications
Predictive maintenance is one of the key applications of AI in this sector. Traditional maintenance strategies often operate on a schedule or are reactive to problems. In contrast, predictive maintenance leverages AI to anticipate issues before they occur. By analyzing data from sensors and machines, AI algorithms can identify signs of wear and tear, predict when a machine is likely to fail, and suggest timely maintenance actions.
Aspect | Traditional Maintenance | AI-Driven Predictive Maintenance |
Approach | Schedule-based or reactive | Proactive and data-driven |
Data Utilization | Limited to manual checks | Extensive use of sensor data |
Failure Prediction | Based on general guidelines | Based on real-time data analysis |
Maintenance Scheduling | Fixed intervals or after failure | Optimized based on equipment needs |
Cost Efficiency | Possible wastage and unplanned costs | Reduced wastage, optimized spending |
Downtime | Potentially high due to unplanned failures | Significantly reduced |
The Importance of Predictive Maintenance
The importance of predictive maintenance in manufacturing cannot be overstated. It’s not just about preventing equipment failures; it’s about fundamentally changing how maintenance is perceived and executed. By predicting when maintenance is needed, manufacturers can schedule interventions at the most opportune times, minimizing disruption to production and extending the lifespan of their machinery. This approach leads to substantial cost savings, improved safety, and higher product quality.
Understanding Predictive Maintenance
Predictive maintenance employs AI to foresee equipment failures, optimizing manufacturing efficiency and minimizing downtime, marking a transformative shift in maintenance practices.
Definition and Explanation of Predictive Maintenance
Predictive maintenance is a proactive maintenance strategy that utilizes data analysis tools and techniques to detect anomalies and predict equipment failure before it occurs. This approach involves continuously monitoring the condition and performance of equipment during regular operation. By leveraging data from sensors, IoT devices, and historical maintenance records, predictive maintenance systems can identify patterns and signs of wear or impending failure. This allows for maintenance to be scheduled at the optimal time, thereby avoiding unexpected downtime and extending the lifespan of machinery.
Comparison with Traditional Maintenance Approaches
Predictive maintenance differs significantly from traditional maintenance approaches in several key aspects:
- Scheduled Maintenance: Traditional methods often rely on a predetermined schedule, leading to unnecessary maintenance activities even when equipment is in good condition.
- Reactive Maintenance: This approach involves waiting for equipment to fail before taking any action. While it avoids unnecessary maintenance, it often leads to increased downtime and higher repair costs.
- Resource Utilization: Traditional maintenance can either overuse or underuse resources. Predictive maintenance optimizes resource allocation by targeting specific areas of need.
- Cost Efficiency: While traditional methods can be costly due to unplanned downtime and over-maintenance, predictive maintenance reduces these costs by anticipating problems before they lead to major breakdowns.
- Equipment Lifespan: Regular, unnecessary maintenance can shorten the lifespan of equipment. Predictive maintenance ensures that machines are serviced only when needed, potentially extending their usability.
- Safety and Risk Management: Predictive maintenance enhances safety by reducing the risk of equipment failure. Traditional methods, especially reactive maintenance, can pose higher risks due to unexpected breakdowns.
The Role of AI in Predictive Maintenance

AI plays a crucial role in predictive maintenance, leveraging data and algorithms to forecast equipment failures, minimizing downtime, and optimizing manufacturing operations.
How AI Algorithms and Machine Learning are Applied
The integration of AI and machine learning (ML) in predictive maintenance marks a significant advancement in manufacturing maintenance strategies. AI algorithms analyze data from various sources, including sensors and historical maintenance records, to detect patterns that might indicate potential equipment failure. Machine learning models, trained on large datasets, can predict future failures by recognizing complex patterns and anomalies that would be impossible for humans to detect. This application of AI and ML transforms raw data into actionable insights, enabling timely maintenance decisions that can prevent costly downtimes and improve overall operational efficiency.
Successful AI Implementation in Predictive Maintenance
Several real-world case studies demonstrate the successful implementation of AI in predictive maintenance:
- Automotive: AI-based predictive maintenance reduced unplanned downtime by 30% and cut maintenance costs by 25%.
- Energy: AI monitored critical equipment, allowing maintenance during low-demand times, boosting productivity, and saving energy.
- Aerospace: AI in aircraft engine monitoring reduced unscheduled maintenance, enhancing flight safety and punctuality.
Benefits of AI-Driven Predictive Maintenance
AI-driven predictive maintenance offers cost savings, reduced downtime, and improved operational efficiency, ensuring equipment reliability and enhanced product quality in manufacturing.
Cost Savings and Efficiency Improvements
The implementation of AI-driven predictive maintenance in manufacturing yields significant benefits in terms of cost savings and operational efficiency:
- Optimized Maintenance Spending: By predicting the exact time when maintenance is needed, AI-driven systems help avoid unnecessary checks and repairs, thereby reducing maintenance costs.
- Increased Equipment Efficiency: Regular, timely maintenance ensures that equipment operates at optimal efficiency, reducing energy usage and wear and tear.
- Resource Optimization: AI predictive maintenance allows for better allocation of human resources, focusing staff on critical tasks rather than routine checks.
- Inventory Management: Predictive analytics can forecast the need for spare parts, enabling more efficient inventory management and avoiding excess stock or shortages.
- Enhanced Production Quality: Well-maintained equipment is less likely to produce defects, leading to an overall improvement in product quality.
Reduction in Unplanned Downtime and Equipment Failures
AI-driven predictive maintenance offers significant advantages, notably by substantially cutting unplanned downtime and equipment failures. It identifies issues before they escalate, decreasing the risk of unexpected breakdowns. Manufacturers can then uphold production schedules, ensuring customer satisfaction. Additionally, it boosts workplace safety by reducing the chances of accidents from equipment malfunctions. Over the long term, this proactive approach extends machinery lifespan by preventing severe wear and tear.
Key Technologies Powering AI Predictive Maintenance
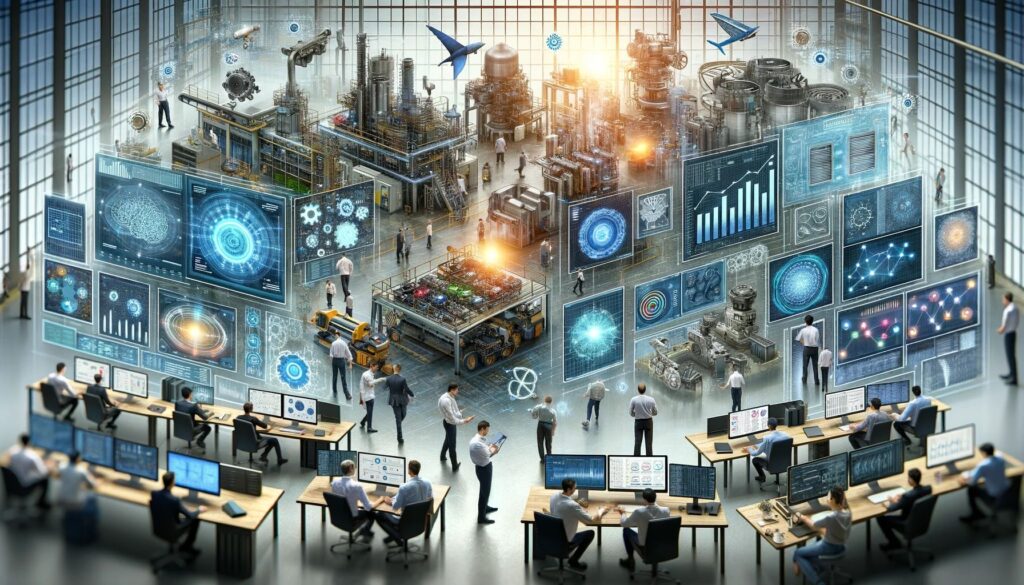
Key technologies driving AI predictive maintenance include machine learning algorithms, IoT sensors, data analytics, and cloud computing, enabling real-time equipment monitoring and preemptive maintenance actions.
IoT and Big Data Analytics in Manufacturing
The successful implementation of AI-driven predictive maintenance in manufacturing relies heavily on the integration of several key technologies:
- Internet of Things (IoT): IoT devices and sensors are fundamental in collecting real-time data from equipment. This includes temperature, vibration, acoustics, and other critical operational parameters.
- Big Data Analytics: The data collected by IoT devices are vast in volume and variety. Big data analytics tools are used to process and analyze this data, uncovering patterns and insights that inform maintenance decisions.
- Cloud Computing: Cloud platforms provide the necessary infrastructure for storing and processing large datasets. They enable scalable, flexible, and secure data management critical for predictive maintenance.
- Edge Computing: In scenarios where real-time data processing is crucial, edge computing allows for data analysis at or near the source of data collection, reducing latency.
- Machine Learning and AI Algorithms: These are at the core of predictive maintenance, utilizing the data gathered to model and predict potential failures.
Overview of Sensors, Data Collection, and Analysis Tools
Sensors and data collection tools are the eyes and ears of predictive maintenance systems, capturing the vital signs of manufacturing equipment. These can include:
- Vibration Sensors: Detect abnormalities in equipment movement, often an early indicator of mechanical issues.
- Temperature Sensors: Monitor changes in temperature, crucial for detecting overheating and other thermal-related problems.
- Acoustic Sensors: Listen for noise anomalies that could indicate issues like leaks or changes in operational efficiency.
- Pressure Sensors: Track pressure levels in hydraulic and pneumatic systems to identify leaks or blockages.
- Optical Sensors: Used for inspections and to detect surface defects or alignment issues.
Challenges and Considerations in Implementing AI
Implementing AI poses challenges such as data privacy, workforce training, and ethical considerations. Addressing these issues is essential for successful integration and responsible AI adoption.
Addressing the Technical and Organizational Challenges
Implementing AI in predictive maintenance is not without its challenges, both technical and organizational. From a technical standpoint, integrating AI requires a robust IT infrastructure capable of handling large volumes of data. There’s also the need for skilled personnel who can manage and interpret AI systems. On the organizational side, a significant challenge is the cultural shift required within the company. Employees need to be trained and made comfortable with AI tools, and processes must be adapted to accommodate new predictive maintenance strategies. Additionally, there’s a need for a strong collaboration between different departments – from IT to the factory floor – to ensure a seamless implementation of AI systems.
Ethical Considerations and Workforce Impact
The ethical considerations and workforce impact of implementing AI in manufacturing also warrant attention. One primary concern is the fear of job displacement. While AI can enhance efficiency, it’s crucial to manage the transition in a way that supports workers, potentially through retraining programs and redeployment into more value-added tasks. There’s also the ethical responsibility to ensure data privacy and security, especially when dealing with sensitive equipment data. The companies must adhere to ethical standards and regulations concerning data use and privacy. Lastly, there’s the aspect of maintaining human oversight. Despite the advancements in AI, human expertise and decision-making remain invaluable, particularly in interpreting complex scenarios that AI might not fully understand.
The Future of Manufacturing with AI
The future of manufacturing with AI promises enhanced efficiency, reduced costs, and improved product quality, revolutionizing production processes and driving innovation in the industry.
Emerging Trends and Potential Future Developments
As AI continues to evolve, several emerging trends and future developments are set to further revolutionize the manufacturing industry:
- Advanced Machine Learning Models: The development of more sophisticated ML models will enable even more accurate predictions and efficient maintenance strategies.
- Increased Automation: Enhanced AI capabilities will lead to greater automation in manufacturing processes, not just in maintenance but across the entire production lifecycle.
- Digital Twins: The use of digital twins – virtual replicas of physical systems – will become more prevalent, allowing for more detailed analysis and simulation.
- Collaborative Robots (Cobots): The integration of AI in robotics will see a rise in cobots, working alongside humans for more efficient and safer operations.
- AI-Driven Quality Control: AI will play a bigger role in quality control, using advanced imaging and data analysis to detect defects with greater accuracy.
- Sustainable Manufacturing: AI will contribute to more sustainable manufacturing practices by optimizing resource use and reducing waste.
How AI is Shaping the Future of the Manufacturing Industry
AI is a transformative force in manufacturing, not just for predictive maintenance but for overall efficiency and adaptability. It optimizes production, enhances product quality, and reduces environmental impact. As AI evolves, it will deeply integrate into manufacturing, creating smarter factories where humans and AI collaborate for unparalleled productivity and innovation. This symbiosis will shape the manufacturing future, fostering an era of unmatched efficiency and growth.
Conclusion
The integration of AI in manufacturing, especially in predictive maintenance, is more than a trend; it’s a transformative shift reshaping the industry. AI-driven predictive maintenance showcases AI’s potential to enhance efficiency, reduce costs, and elevate manufacturing operations. The real-world benefits underline its profound impact, making processes proactive and efficient. The future holds even more potential with advanced machine learning, digital twins, and collaborative robots, promising sustainable and intelligent manufacturing practices. To ensure success, we must navigate challenges thoughtfully, leveraging human expertise alongside AI innovation for the industry’s full potential.