The integration of Artificial Intelligence (AI) in healthcare has ushered in an era of unprecedented advancements, particularly in the field of medical diagnostics. AI’s contribution to healthcare is not just a fleeting trend but a fundamental shift in how medical care is delivered and experienced. At the core of this transformation is the ability of AI systems to process vast amounts of data, recognize patterns, and provide insights that were previously unattainable with traditional methods.
Evolution of Medical Technology with AI
The journey of medical technology has been remarkable, from the advent of the stethoscope to the introduction of AI. In the past, diagnostics relied heavily on the manual interpretation of symptoms and test results, which, while effective, had limitations in terms of accuracy and efficiency. The integration of AI into this realm has changed the game entirely. AI algorithms, powered by machine learning and deep learning, can analyze complex medical data at an unprecedented speed and with a higher degree of precision.
This evolution is not just about speed and accuracy; it’s about the holistic improvement of patient care. AI in diagnostics extends beyond identifying diseases; it includes predicting patient outcomes, suggesting personalized treatment plans, and even foreseeing potential complications before they arise. This proactive approach in healthcare is pivotal in enhancing patient outcomes and reducing healthcare costs.
AI’s Role in Diagnosing Diseases
AI’s role in diagnosing diseases is multifaceted. It includes analyzing electronic health records (EHRs) to identify disease patterns, interpreting medical images, and even using genetic information to predict the likelihood of diseases. For instance, AI systems are being used to detect cancers more accurately in imaging tests like MRIs and CT scans, often identifying subtle changes that are imperceptible to the human eye.
Moreover, AI’s capability to learn and improve over time means that these systems become more sophisticated with each diagnosis. They continuously learn from new data, enabling them to make more accurate predictions and diagnoses in the future.
Aspect | Traditional Methods | AI-Enhanced Methods |
Speed of Diagnosis | Variable, often slower | Rapid and efficient |
Accuracy | Dependent on individual expertise | High, with continuous learning |
Data Analysis | Limited by human capacity | Vast data processing capabilities |
Personalization | Generalized treatments | Tailored treatment plans |
Predictive Analysis | Mostly manual forecasting | Automated, data-driven predictions |
Cost Efficiency | Higher due to longer diagnostic processes | Reduced due to efficiency and precision |
The Mechanics of AI in Medical Diagnostics
AI’s remarkable ability to transform healthcare, particularly in diagnostics, hinges on its sophisticated algorithms and advanced data processing capabilities. These AI systems, primarily powered by machine learning and deep learning techniques, are redefining how medical professionals approach diagnostics.
How AI Algorithms Work in Diagnosing Diseases
AI in medical diagnostics operates through a process of learning from large datasets. These datasets can include medical images, patient health records, lab results, and even genetic information. Machine learning algorithms, a subset of AI, are trained using this data to recognize patterns and anomalies that might indicate a specific health condition.
Deep learning, a more advanced form of machine learning, takes this a step further. It involves neural networks – a series of algorithms modeled on the human brain’s structure and function – to interpret complex data. For instance, in image-based diagnostics, deep learning algorithms can analyze thousands of radiological images to learn how to identify signs of diseases such as tumors or fractures.
Examples of AI Implementations in Diagnostic Scenarios
- Cancer Detection: AI systems have shown remarkable proficiency in detecting cancers, such as breast and lung cancer, in early stages. For example, AI algorithms used in mammography can spot subtle signs of breast cancer with higher accuracy than traditional methods.
- Cardiovascular Disease Prediction: AI tools analyze EHRs and imaging data to predict cardiovascular risks. These systems can detect minute changes in ECGs or heart MRIs, identifying patients at risk of heart attacks before they occur.
- Neurological Disorder Diagnosis: In neurology, AI is used to diagnose conditions like Alzheimer’s disease by analyzing patterns in brain imaging data. This helps in early diagnosis, crucial for managing such conditions.
- Diabetic Retinopathy Screening: AI algorithms have been effectively used to screen for diabetic retinopathy, a condition that can lead to blindness. By analyzing retinal images, AI can detect this condition at an early stage, enabling timely treatment.
Bridging AI and Human Expertise
While AI brings efficiency and accuracy, it is not about replacing human doctors but rather augmenting their capabilities. The ideal scenario is a collaborative one where AI algorithms provide insights and doctors use their expertise for final decision-making. This synergy ensures that the diagnostics are not just data-driven but also incorporate the essential human elements of empathy and ethical judgment.
The Continuous Evolution of AI in Diagnostics
As AI technology evolves, so does its application in medical diagnostics. Continuous research and development are leading to more sophisticated and precise algorithms. The future holds the promise of AI systems that can diagnose a broader range of conditions with even greater accuracy, making a significant impact on global healthcare.
AI’s Impact on Personalized Medicine
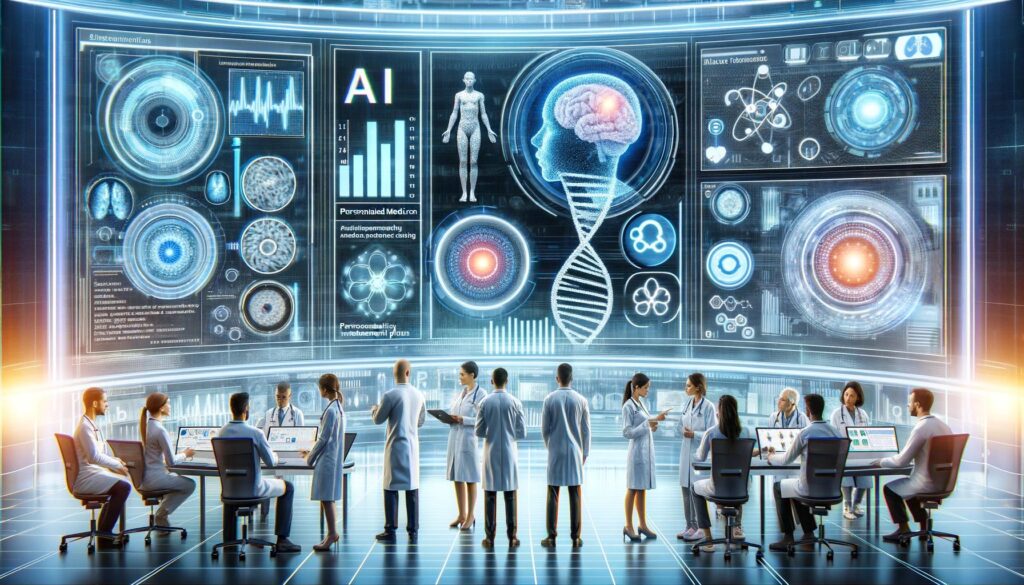
The advent of AI in healthcare is not only revolutionizing diagnostics but is also playing a crucial role in the emergence of personalized medicine. This approach tailors medical treatment to the individual characteristics of each patient, and AI is at the forefront of making this a reality.
Tailoring Treatments Based on AI-Driven Data Analysis
Personalized medicine, driven by AI, relies heavily on data analysis. AI systems can process and analyze vast amounts of patient data, including genetic information, lifestyle factors, and previous health records, to identify the most effective treatment options for individual patients. This data-driven approach enables healthcare providers to move away from the ‘one-size-fits-all’ model to more personalized care.
The Future of Patient Care with Personalized Approaches
The future of patient care with AI-driven personalized medicine is bright. It promises more effective treatment plans, reduced side effects, and overall better patient outcomes. Personalized medicine also has the potential to reduce healthcare costs by eliminating the trial-and-error approach of finding the right treatment.
The integration of AI in personalized medicine is not just about treatment but also prevention. Predictive analytics, a key feature of AI, can identify risk factors for diseases, allowing for early intervention and preventive measures tailored to individual risk profiles.
Challenges and Opportunities in Personalized Medicine
While the prospects are promising, the road to fully integrated personalized medicine is not without challenges. These include managing and analyzing the vast amounts of data required, ensuring patient privacy, and addressing ethical concerns related to AI decision-making.
Despite these challenges, the opportunities presented by AI in personalized medicine are immense. It has the potential to transform healthcare from a reactive to a proactive field, where prevention and early intervention become as important as treatment, leading to a more efficient and effective healthcare system.
Improving Diagnostic Accuracy with AI
One of the most significant contributions of AI in healthcare is its ability to enhance the accuracy of medical diagnostics. This advancement not only improves patient outcomes but also plays a pivotal role in the overall efficiency and effectiveness of healthcare systems.
Statistics and Examples of Increased Accuracy in Diagnoses
The integration of AI in medical diagnostics has led to a noticeable improvement in diagnostic accuracy. For example, studies have shown that AI algorithms can identify certain types of cancers, such as skin cancer, with a level of accuracy comparable to, and sometimes surpassing, experienced dermatologists. Similarly, in radiology, AI has demonstrated a high degree of precision in detecting abnormalities in imaging scans, reducing the likelihood of misdiagnosis.
These improvements are not just theoretical. They translate into real-world benefits, such as earlier detection of diseases, which is often crucial for successful treatment. By accurately diagnosing conditions at an early stage, AI can significantly improve the prognosis for many patients.
Reducing Human Error in Medical Assessments
- Human error is inevitable in healthcare, especially in diagnostics due to subjective interpretation of symptoms and test results.
- AI mitigates diagnostic errors by offering objective, data-driven insights.
- AI systems excel at processing and analyzing medical data with consistency and precision, surpassing human capabilities, especially when time and resources are limited.
- AI serves as a valuable second opinion, offering additional perspectives in complex cases.
- The collaboration between AI and human expertise results in more accurate diagnoses and improved patient care.
The Role of AI in Continuous Learning and Improvement
A unique feature of AI in diagnostics is its ability to continuously learn and improve. As AI systems are exposed to more data, they can refine their algorithms, leading to increasingly accurate diagnoses over time. This aspect of continuous learning is crucial in a field where new medical discoveries and technologies are constantly emerging.
AI in Pathology and Imaging
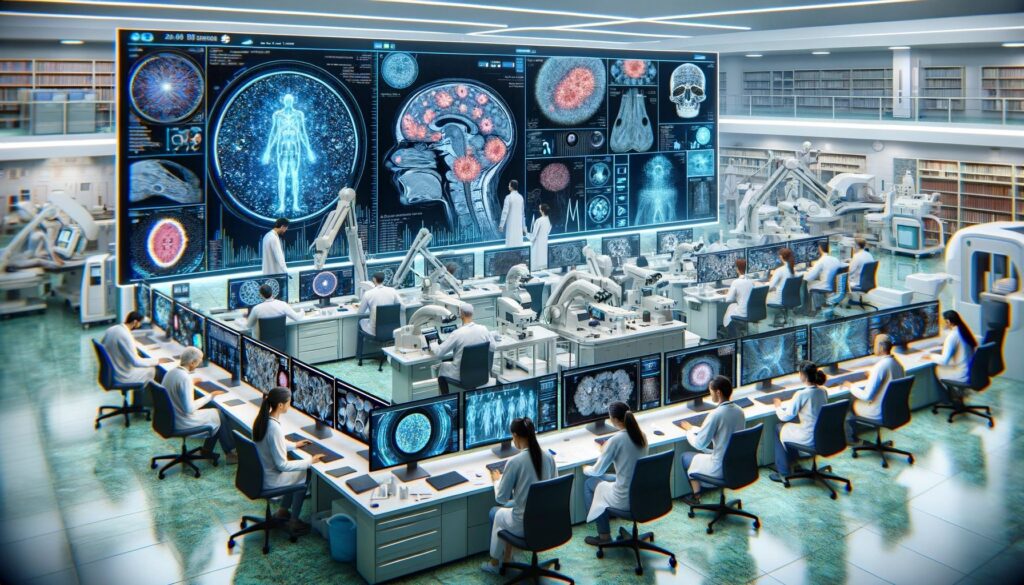
The application of Artificial Intelligence in pathology and medical imaging represents a significant leap forward in diagnosing and understanding various diseases. AI’s ability to analyze and interpret medical images is changing the landscape of these fields, offering more precision and aiding in quicker decision-making processes.
The Role of AI in Interpreting Medical Images
In medical imaging, such as MRI, CT scans, and X-rays, AI algorithms play a crucial role in detecting and interpreting abnormalities. These algorithms are trained to recognize patterns indicative of specific conditions, often identifying subtle changes that may be missed by the human eye. For instance, in radiology, AI can detect nuances in imaging that signify early stages of diseases like cancer, allowing for earlier intervention.
AI’s contribution to pathology is equally transformative. In digital pathology, where slides of tissues are analyzed to understand diseases, AI can rapidly process and analyze vast numbers of images, providing pathologists with detailed insights that assist in making accurate diagnoses. This capability is especially crucial in complex cases where the human eye might miss subtle, yet significant, details.
Enhancements in Pathology with AI Technologies
AI technologies have brought about enhancements in pathology by automating routine tasks, allowing pathologists to focus on more complex aspects of diagnosis. For example, AI can be used to count cells, identify cell types, or detect abnormalities in tissue samples, tasks that are time-consuming and prone to human error when done manually.
AI-Powered Imaging: Beyond Diagnosis
Beyond diagnostic capabilities, AI-powered imaging is also advancing research in various medical fields. It enables researchers to discover new biomarkers and understand disease mechanisms more profoundly. The insights gained from AI analysis of medical images are contributing to the development of new drugs and treatment methods, further enhancing patient care.
Ethical Considerations and Patient Privacy
As AI continues to make inroads into healthcare, particularly in diagnostics, it brings with it a host of ethical considerations and concerns regarding patient privacy. Navigating these challenges is crucial for maintaining trust and integrity in healthcare.
Balancing Technological Advances with Ethical Concerns
- Informed Consent: Ensuring patients are fully informed about how AI is used in their diagnosis and treatment. Patients should understand what data is being used, how it is processed, and the implications of AI-driven decisions.
- Bias and Fairness: Addressing potential biases in AI algorithms. AI systems are only as unbiased as the data they are trained on. It’s essential to ensure diverse datasets to avoid inadvertently perpetuating existing biases in healthcare.
- Transparency: Maintaining transparency in AI algorithms. While complete transparency might be challenging due to the complexity of AI models, it’s vital to ensure that the basis of AI decisions can be understood and trusted by medical professionals.
- Accountability: Establishing clear accountability for AI-driven decisions. It should be clear who is responsible for the outcomes of an AI-assisted diagnosis – the AI developers, the healthcare providers, or a combination of both.
- Ethical Deployment: Ensuring the ethical deployment of AI. This includes considering the impact on healthcare jobs, patient-doctor relationships, and the overall healthcare system.
Safeguarding Patient Data in the Age of AI
The use of AI in healthcare presents significant privacy and security challenges due to the handling of sensitive patient data. To address these concerns, healthcare systems must implement robust data protection measures, including encryption, anonymization, and strict access controls. Compliance with regulations like GDPR and HIPAA is essential to ensure patient data remains confidential.
Furthermore, ongoing collaboration between AI developers, healthcare providers, and regulators is crucial to address emerging privacy issues. By prioritizing patient privacy and ethical considerations, the healthcare sector can harness AI’s benefits while maintaining patient trust.
Conclusion
The integration of AI in healthcare, especially in diagnostics, marks a paradigm shift in health management. This shift promises increased accuracy, efficiency, and personalization. AI’s involvement in diagnostics, pathology, imaging, and personalized medicine enhances patient outcomes and reduces costs. Ethical, legal, and privacy concerns must be addressed to ensure responsible innovation and data security. The collaboration between AI and human expertise holds the key to a more accessible and effective healthcare future. Embracing this transformative journey will create a patient-centric, advanced healthcare system.